Researcher John Paparrizos Wins ACM SIGKDD Dissertation Distinction
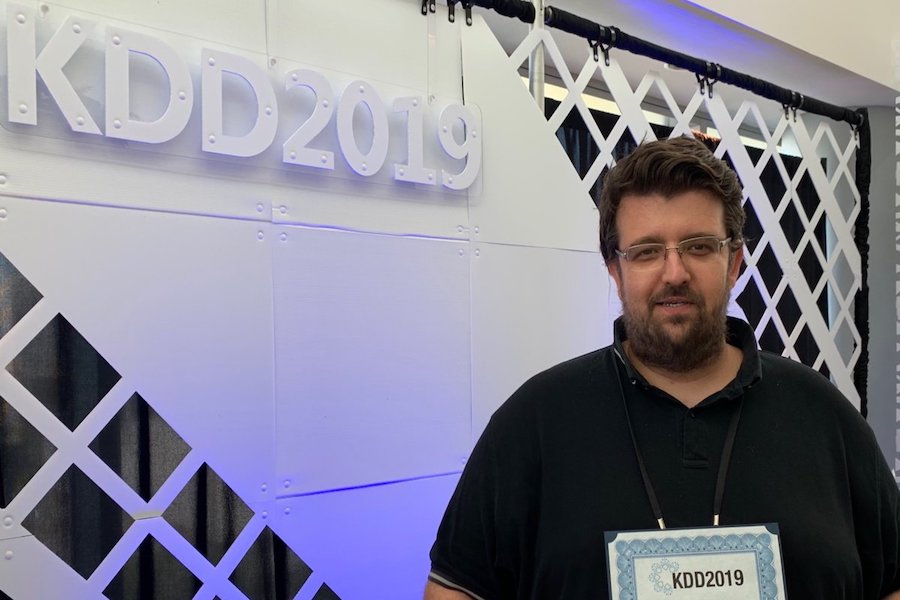
With more and more data pouring in from scientific collaborations, the internet, and sensored environments and machines, new systems and algorithms are needed to make sense of all that information. Many of these data streams take the form of time series, with values collected sequentially over periods of time, such as hourly weather data or stock market prices. But while time series are a very common format, researchers still lack the standards needed to automate their analysis.
As a PhD student at Columbia University and a postdoctoral researcher at the University of Chicago, John Paparrizos has worked to address this challenge. At this month’s 2019 ACM SIGKDD conference in Alaska, Paparrizos’ thesis, “Fast, Scalable, and Accurate Algorithms for Time-Series Analysis,” received an Honorable Mention for KDD’s Doctoral Dissertation Award.
Paparrizos’ dissertation describes a new set of algorithms and automated methods for analyzing time-series data, regardless of their domain.
“The good thing is that currently we have the technological maturity to collect and store this data,” Paparrizos said. “We have different types of sensors for collecting data from natural processes and human-made artifacts, we have the computational infrastructure to store them, and we have large-scale dataflow systems to process them. But the fact is all of these systems, as well as most of the methods they support, have been designed for essentially static data. With the rapid growth of Internet-of-Things data volumes, we need to support applications for data that evolve over time.”
Typically, researchers analyzing time series need to do the same set of analytic tasks as in other domains, such as similarity search, classification, and clustering. But due to several challenges, such as the broad ranges of domains that generate time series and the high-dimensionality of datasets that can have millions of time points, the representations required for these analyses are usually created from scratch, one project or application at a time.
“What we were saying was, can we do something better than that? Can we essentially automate the process of constructing representations that preserve crucial characteristics to support time-series analytics?,” Paparrizos said. “It’s not sustainable to have Ph.D. students working for five years in order to achieve these things again and again.”
Experiments in Paparrizos’ dissertation showed that the proposed methods achieve state-of-the-art performance on over 80+ different time-series datasets, though much more efficiently than prior work. That’s useful not only for saving scientists’ time in the future, but also for developing analytic systems capable of running on limited computational resources, which will be critical for the next wave of Internet of Things and edge computing applications.
The thesis also describes methods for two new scientific contexts. In one, Paparrizos helped create a model that predicts which scientific concepts will have long-term impact, to help guide the decisions of funding agencies. Another project created a system that detects when people search for symptoms that may be predictive of serious diseases such as pancreatic cancer, which could trigger warnings to seek medical testing.
At UChicago, Paparrizos continues his thesis work by integrating the methods into databases, so that users can perform their analyses without moving these large datasets to external software. He’s also expanding his work for multivariate time series and to exploit alternative approaches, such as neural networks. Last year, he received a fellowship from data services company NetApp to create new methods that enable the analysis of compressed, large-scale data.
“Companies and scientists are now measuring multiple things at the same time, and they want to perform analysis over multiple different sensors, which will require significant changes in current approaches,” Paparrizos said.