Aditi Raghunathan (Stanford) - Rethinking the Role of Data in Robust Machine Learning
Rethinking the Role of Data in Robust Machine Learning
Despite notable successes on several carefully controlled benchmarks, current machine learning (ML) systems are remarkably brittle, raising serious concerns about their deployment in safety-critical applications like self-driving cars and predictive healthcare. In this talk, I discuss fundamental obstacles to building robust ML systems and develop principled approaches that form the foundations of robust ML. I will focus on two settings where standard ML models degrade substantially: adversarial attacks on test inputs, and presence of spurious correlations like image backgrounds. I will demonstrate the need to question common assumptions in ML, particularly about the role of training data. On the one hand, I will describe how and why naively using more data can surprisingly hurt performance in these robustness settings. On the other hand, I will show that unlabeled data, when harnessed in the right fashion, is extremely beneficial and enables state-of-the-art robustness. In closing, I will discuss how to build on the foundations of robust ML and achieve wide-ranging robustness in various domains including natural language processing and vision.
Host: Ben Zhao
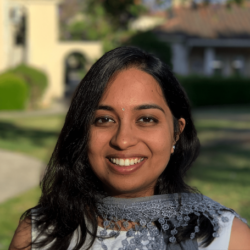
Aditi Raghunathan
Aditi Raghunathan is a fifth year PhD student at Stanford University advised by Percy Liang. She is interested in building robust machine learning systems with guarantees for trustworthy real-world deployment. Her research in robustness has been recognized by a Google PhD Fellowship in Machine Learning and the Open Philanthropy AI Fellowship. Among other honors, she is also the recipient of the Anita Borg Memorial Scholarship and the Stanford School of Engineering Fellowship.