New EAGER Grant to Asst. Prof. Eric Jonas Will Explore ML for Quantum Spectrometry
The vision of the automated laboratory – where robotics and autonomous devices conduct high-throughput experiments with limited human intervention – promises to dramatically accelerate and streamline the process of scientific discovery. But obstacles remain where expensive, complex instruments and manual data analysis are required to gather data and interpret results.
Nuclear magnetic resonance (NMR) spectroscopy, a chemistry technique for determining the structure of an organic compound, is one such speed bump. High-resolution NMR requires room-sized machines that cost millions of dollars, measure one sample at a time, and generate data that requires human experts to interpret. New “zero-field” NMR technologies coming to market may solve the cost and scale problem, but produce data that’s currently impossible for scientists to decode.
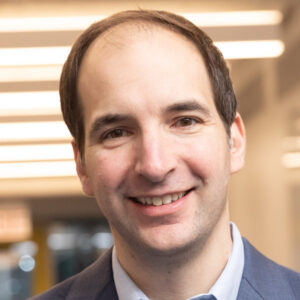
With a new Early-Concept Grants for Exploratory Research (EAGER) award from the National Science Foundation, UChicago CS Assistant Professor Eric Jonas will see if machine learning can overcome these challenges. In collaboration with Ashok Ajoy from the University of California, Berkeley, Jonas will create a model for automatically interpreting the data from the new class of quantum spectroscopy sensors. The work could help enable laboratory automation for the faster discovery of small molecules useful as new drugs and materials.
“The hope with these new sensors is, because these things are small, you can measure 100 or 1000 chemicals at once, instead of one at a time,” Jonas said. “But that creates a last mile problem, where the robots analyze a bunch of chemicals, but they produce all this spectroscopic data that no one knows how to interpret.”
The new project builds upon previous work by Jonas using deep imitation learning to read NMR spectra from traditional instruments. Spectroscopy data – clusters of peaks corresponding to the atoms and bonds present in a molecule – proved a natural fit for machine learning, which could learn from millions of real and synthetic readings to predict the most likely molecular structures from a given spectrum.
But the new zero-field sensors produce a different kind of spectrum data, which not only renders the traditional hand-reading methods obsolete, it requires new machine learning approaches to decode. And without human interpretation to compare against, the benchmarks for success are even harder to judge, Jonas said.
“Normal NMR is like every atom telling you its name,” Jonas said. “With this new NMR, every atom is just telling you who its friends are. So it becomes harder to disentangle this information, with just that raw coupling data.”
Solving these issues would not only make the zero-field NMR technology more viable for laboratory use, it could also transform how chemists understand and use these methods. With high-throughput measurements, scientists can more rapidly test new ways of interrogating compounds for their structural information. In turn, these new approaches could unlock new surveys of small molecules, the mapping of unknown molecular pathways, real-time monitoring of reactions, and other frontiers of chemistry.
“The hope is that this sort of non-destructive spectroscopic measurement is going to become more ubiquitous more broadly,” Jonas said. “I think we’re going to get to the point where we really deeply understand what we need to know about a spin system to then say something interesting about the structure of the molecule. Down the road, we may understand these general principles, and we’ll know exactly how to stimulate each molecule to get it to give up its secrets.”
[NMR Photo: Andrea Starr | Pacific Northwest National Laboratory]