Yuanhao Wang (Princeton)- Is RLHF more difficult than standard RL? A view from reductions
Reinforcement learning from Human Feedback (RLHF) learns from preference signals, while standard Reinforcement Learning (RL) directly learns from reward signals. Preferences arguably contain less information than rewards, which makes preference-based RL seemingly more difficult. This paper theoretically proves that, for a wide range of preference models, we can solve preference-based RL directly using existing algorithms and techniques for reward-based RL, with small or no extra costs. Specifically, (1) for preferences that are drawn from reward-based probabilistic models, we reduce the problem to robust reward-based RL that can tolerate small errors in rewards; (2) for general arbitrary preferences where the objective is to find the von Neumann winner, we reduce the problem to multiagent reward-based RL which finds Nash equilibria for factored Markov games under a restricted set of policies. The latter case can be further reduced to adversarial MDP when preferences only depend on the final state. We instantiate all reward-based RL subroutines by concrete provable algorithms, and apply our theory to a large class of models including tabular MDPs and MDPs with generic function approximation. We further provide guarantees when K-wise comparisons are available.
Speakers
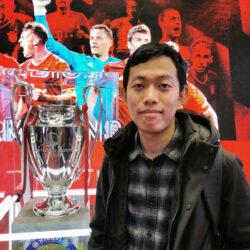
Yuanhao Wang
Yuanhao Wang is a fourth-year PhD student at the Computer Science Department of Princeton University. He is advised by Chi Jin. Prior to Princeton, he received his bachelor’s degree in Computer Science from Yao Class at Tsinghua University. His research interests include reinforcement learning theory, learning in games and minimax optimization. He has received the best paper award in the ICLR 2022 workshop on Gamification and Multiagent Solutions.