$3.25m DOE Grant Funds UChicago/Argonne Research on AI Models of Physics Simulations
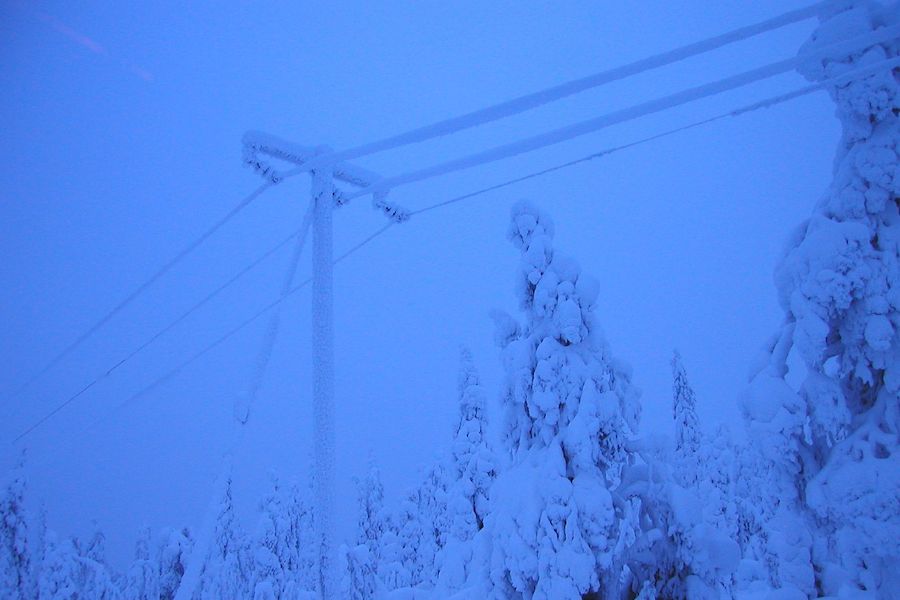
As the world’s climate changes, extreme events considered “once-in-a-century” emergencies appear much more frequently than the name suggests. From prolonged cold snaps and heat waves to infrastructure collapses such as floods and blackouts, these rare and hard-to-predict events challenge governments and industries in their preparation for worst case scenarios. But a new collaboration between University of Chicago and Argonne National Laboratory researchers will apply artificial intelligence to accelerate the scientific simulation of complex physical systems, with the potential to more accurately determine the probability of these extremes.
The project, funded through a $3.25 million grant from the U.S. Department of Energy (DOE), will explore the fundamentals of “surrogate models” — simplified models built using artificial intelligence that speed up the complex scientific models for climate, energy infrastructure, and other systems. By allowing researchers to run many more simulations in the same amount of time, these surrogates enable better quantification of the risk of extreme events, the use of computer modeling in rapid decision-making, and other advantages.
But questions remain about whether these new models adequately represent the original versions, which solve complicated mathematical equations to recreate physical processes. The project will also draw from other AI applications to find new ways of creating and training surrogate models.
“We will systematically explore different families of models and try to understand which characteristics are most predictive of whether or not we’ll be able to build a good surrogate,” said Rebecca Willett, professor of statistics and computer science at the University of Chicago and principal investigator on the new project. “We’re also taking ideas that have been developed and explored in the context of natural language processing or computer vision and incorporating those ideas into surrogates, hopefully by incorporating additional physics information to make them more robust to small amounts of data or extreme events.”
Joining Willett in this collaboration are assistant professors Yuehaw Khoo and Daniel Sanz-Alonso of the UChicago Department of Statistics, assistant professor of mathematics Dana Mendelson, Argonne Assistant Computational Statistician Julie Bessac, and Mihai Anitescu, Senior Computational Mathematician at Argonne and part-time professor of statistics at UChicago. Willett, Anitescu, Khoo, and Sanz-Alonso are also part of the Committee on Computational and Applied Mathematics (CCAM). The award was part of $16 million awarded by the DOE to five groups studying data-intensive scientific machine learning and analysis.
“The University of Chicago is committed to working with our national laboratory partners on large-scale problems like climate change, which can only be addressed through these types of scientific collaborations across disciplines and institutions,” said Juan de Pablo, UChicago Vice President for National Laboratories, Science Strategy, Innovation, and Global Initiatives. “AI technology can play a key role in addressing challenging issues that have global impact.”
Faster Simulations, Better Risk Assessment
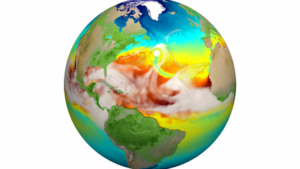
The most advanced computer models for studying the Earth’s climate, fluid dynamics, or the operation of the national power grid run on partial differential equations (PDEs), mathematical equations used to describe physical laws. But these equations are difficult to solve, and it may require hundreds of hours on the world’s most powerful supercomputers to run a single simulation.
Surrogate models offer an AI-fueled alternative. Instead of running the model, scientists feed the initial conditions and results of past model runs as data into a machine learning mode, such as a neural network, which learns to replicate the predictions of the full supercomputer simulation. Once trained, these AI models produce results many times faster than the original, allowing scientists to better characterize the range of possible outcomes through repeated trials.
“The use of surrogate models in the context of data-centric problems in scientific applications is important, because most of the tasks involve repeated evaluations of the models,” said Sanz-Alonso. “Each time you need to evaluate the model, it’s computationally costly, and in some applications, you need to evaluate the model millions of times, so it is particularly important to be able to do it cheaply.”
More runs also allow for better assessments of low-probability, high-impact events that may only turn up in a minute percentage of simulations. The original model, run only a handful of times due to computational limitations, may miss these rare occurrences. But a surrogate model, run hundreds or thousands of times, could spot them and accurately characterize their frequency.
With that information, authorities could better prepare for the “black swan” events that produce tremendous costs and casualties, Anitescu said. Infrastructure designed to survive “once-in-a-century” events will have a more reliable estimate of what that means, while the frequency of extreme climate events could be better understood and anticipated.
“It’s more useful for decision making, particularly when you care about getting the risk of extreme events properly,” Anitescu said. “But we have to be able to model them faster, because things are changing. If we’re not able to tell people what to expect from real rare events, like floods and hurricanes, and how much does it really cost to build on a coast, somebody is going to go bankrupt, either the people who build the houses or the government.”
The UChicago/Argonne team is well suited to shoulder the multidisciplinary breadth of the project, which spans from mathematical foundations to cutting edge data and computer science concepts in artificial intelligence to modeling applications in many different scientific fields. The research was also originally initiated by an “AI + Science” seed grant from the UChicago Joint Task Force Initiative (JTFI), which supports collaborations between UChicago, Argonne, and Fermilab.
”Collaborations between UChicago and the national labs allow us to leverage broad sets of skills across disciplines to address these challenges,” Willett said. “The seed funding provided by the JTFI in the area of AI for Science has been critical in terms of encouraging us to form partnerships and define grand challenges that can only be addressed through large collaborative efforts.”