Bryon Aragam (UChicago Booth) - A General Framework for Learning DAGs with NO TEARS
A General Framework for Learning DAGs with NO TEARS
Interpretability and causality have been acknowledged as key ingredients to the success and evolution of modern machine learning systems. Graphical models, and more specifically directed acyclic graphs (DAGs, also known as Bayesian networks), are an established tool for learning and representing interpretable causal models. Unfortunately, estimating the structure of DAGs from data is a notoriously difficult problem, and as a result existing approaches rely on various local heuristics for enforcing the acyclicity constraint. In this talk, we introduce a fundamentally different strategy: We formulate the structure learning problem as a purely continuous optimization problem that avoids this combinatorial constraint entirely. This optimization problem can be efficiently solved by standard numerical algorithms, avoiding handcrafted algorithms which also makes implementation particularly easy. As a result, we obtain a general framework for learning parametric, nonparametric, and dynamic DAG models that includes GLMs, additive noise models, and index models as special cases.
Joint work with Xun Zheng, Chen Dan, Pradeep Ravikumar, and Eric P. Xing.
Host: Eric Jonas
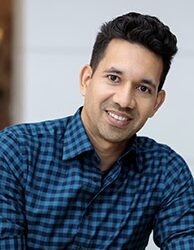
Bryon Aragam
Bryon Aragam is an Assistant Professor and Topel Faculty Scholar in the Booth School of Business at the University of Chicago. His research interests include statistical machine learning, nonparametric statistics, and optimization. He is also involved with developing open-source software and solving problems in interpretability, ethics, and fairness in artificial intelligence.
Prior to joining the University of Chicago, Bryon was a project scientist and postdoctoral researcher in the Machine Learning Department at Carnegie Mellon University. He completed his PhD in Statistics and a Masters in Applied Mathematics at UCLA. He has also served as a data science consultant for technology and marketing firms, where he has worked on problems in survey design and methodology, ranking, customer retention, and logistics