Michael Maire, An Architect of Deep Learning, Joins CS from TTIC
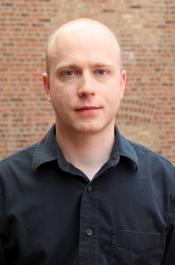
In 2012, the ImageNet computer vision competition was the breakthrough moment for a new top contender for artificial intelligence applications: deep learning. The surprise victory of this approach over traditional machine learning methods revived interest in deep convolutional neural networks (CNNs), a decades-old concept rejuvenated by big data and more powerful computing. Since then, researchers have further explored these models for visual tasks such as image classification and face recognition, and expanded their use into robotics, natural language processing, computational biology, and other areas.
At the time of the ImageNet competition, new UChicago CS assistant professor Michael Maire was a postdoctoral researcher in the Caltech Computer Vision Lab. His work since, including 4 years at the Toyota Technological Institute at Chicago, has focused on the architectures of these CNNs, studying their underlying structure and the modifications that will help apply deep learning to new, more complicated applications.
“The goal is not just to focus on efficiency improvements,” Maire said, “but to get some understanding of what design details matter in the neural network architecture itself and to put engineering and design effort into that architecture, so that we can get new capabilities out of the neural network and train it to accomplish more complex tasks.”
Deep neural networks were originally inspired by the anatomy of the human brain, where information is processed by intricately connected systems of neurons. In a computational neural network, multiple connected layers take in raw input data, such as the pixels of an image, and gradually transform the information, identifying features and eventually assigning a label, such as determining whether the image contains a human or a dog.
Computer scientists like Maire have explored different sizes and structures for these networks, with the “deep” in deep learning typically referring to networks with tens or even hundreds of layers. Maire’s work also looks at how these networks can be trained, including on data with little or no human labeling, and higher-order visual capabilities, such as understanding the detailed composition of scenes containing many objects. These functions will be particularly useful as engineers further develop autonomous vehicles, robotics, and other technologies that rely upon advanced computer vision.
“The goal is human-level understanding and perception of the visual environment,” Maire said. “We’re moving towards models that learn something about the environment when presented with new objects, and that are capable of making decisions on the fly.”
In addition to studying the architecture of CNNs, Maire also contributes to the data they are tested with through his work on the COCO (Common Objects in Context) dataset. Comprised of over 330,000 images of complex everyday scenes, COCO provides a target for scientists to test new methods in object detection, captioning, and segmentation. A workshop and challenges take place each year, alternating between the ICCV and ECCV conferences.
As a nearby neighbor at TTIC, Maire already worked with UChicago graduate students. Last year, he published a paper with UChicago CS PhD student Gustav Larsson on building a network to automatically colorize images. As data for this task can be collected automatically, without human labeling effort, the network can learn in a self-supervised manner. In addition, colorization serves as a proxy task for larger goals of scene understanding; naming a plausible color for an object is linked to understanding its identity. This fall, Maire will teach a computer vision course that will alternate between the university and TTIC.
For more on Maire’s research, visit his webpage at http://ttic.uchicago.edu/~mmaire/