Steve Hanneke (TTIC) - Machine Learning Theory Beyond Uniform Convergence
Machine Learning Theory Beyond Uniform Convergence
For over 50 years, statistical learning theory has developed largely based on the “uniform convergence” principle: for any model that is not too complex, a learner's performance on the training data is a good indicator of its expected performance on future yet-unseen examples. Uniform convergence motivates a natural learning strategy, known as Empirical Risk Minimization (ERM), where an algorithm simply optimizes over the model parameters to fit the training data as well as possible. While these ideas have led to powerful and beautiful theories, recent works have revealed limitations of uniform convergence for understanding the performance of certain learning algorithms, and of ERM as a viable approach to achieving certain desirable performance criteria. These observations reveal a need for new approaches to the design and analysis of machine learning algorithms. In this talk, I present a few examples from my recent work.
As a first example, we consider rates of convergence of an algorithm's generalization error as a function of number of training examples. Our work provides a complete characterization of the optimal rates of convergence. However, the rates achievable by general ERM learners can be suboptimal by an arbitrarily large gap. Rather than uniform convergence, our optimal learner is based on solutions of a game-theoretic interpretation of the learning problem.
As another example, it is known that many learning algorithms are unstable, in the sense that even if they are correct on a given test example, an adversary can change the learner's prediction by perturbing the example an imperceptible amount. Our work reveals that the natural ERM approach to addressing this, known as “adversarial training”, can fail spectacularly. However, approaching the problem from a different perspective, not relying on uniform convergence, we propose a new learning algorithm that is provably robust to such adversarial attacks.
I will conclude with some ongoing work toward a general theory of data-dependent generalization bounds, yielding performance guarantees for certain learning algorithms where there is no corresponding bounded-capacity hypothesis class to which traditional uniform convergence arguments could be applied.
Based on various joint works with Olivier Bousquet, Omar Montasser, Shay Moran, Nathan Srebro, Ramon van Handel, and Amir Yehudayoff.
Host: Rebecca Willett
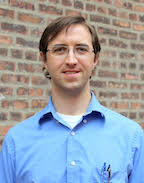
Steve Hanneke
Steve Hanneke is a Research Assistant Professor at the Toyota Technological Institute at Chicago. His research explores the theory of machine learning, with a focus on reducing the number of training examples sufficient for learning. His work develops new approaches to supervised, semi-supervised, active, and transfer learning, and also revisits the basic probabilistic assumptions at the foundation of learning theory. Steve earned a Bachelor of Science degree in Computer Science from UIUC in 2005 and a Ph.D. in Machine Learning from Carnegie Mellon University in 2009 with a dissertation on the theoretical foundations of active learning.