What’s Real and What’s Not? Watermarking to Identify AI-Generated Text
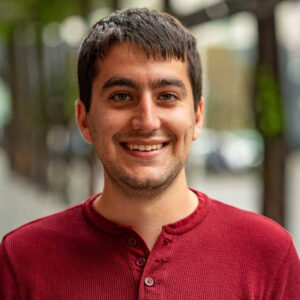
AI is becoming an increasingly ubiquitous technology, but its rise in popularity does not come without problems. For one, it is becoming increasingly difficult to tell what is human versus AI-generated, which raises a slew of security concerns. One of the main goals of a new field of study — watermarking language models — involves embedding detectable signals within the outputs of language models such as ChatGPT, similar to how artists publish their photos with watermarks to prevent copyright violations. Google, for example, now uses watermarking on their flagship AI language model, Gemini, to enable detectingAI-generated text. In their recent paper, Watermarking Language Models for Many Adaptive Users, Assistant Professor Aloni Cohen, post-doctorate Alexander Hoover, and second-year PhD student Gabe Schoenbach extend the theory of watermarking language models.
The researchers became interested in watermarking during their reading group last summer. There, they came across undetectable watermarks, which embed a signal into the outputs of a language model without noticeably changing the quality of the text it produces. In their paper, Cohen, Hoover, and Schoenbach introduce a new property – adaptive robustness – and prove that existing watermarking schemes are adaptively robust. They also build an undetectable, adaptively robust “message-embedding” watermarking scheme that can be used to identify the user who generated the text.
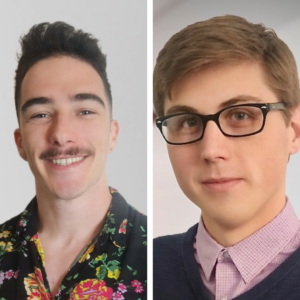
“One important property of a watermark is its robustness,” Schoenbach said. “If you’re embedding a signal into a paragraph of text, it would be nice to have a guarantee that even if someone changes, say, 10% of the text, the signal will still persist. Prior to our paper, there were several watermarking schemes that provided this guarantee, though only in the narrow case where someone prompts the language model just once. But in the real world, people prompt ChatGPT tons of times! They might see many outputs before they stitch some text together, editing here and there to produce a final result. Our idea was that watermarks should persist even when a user can prompt the model multiple times, adaptively changing their prompts depending on the text that they see. Our paper is the first to define this stronger robustness property, and we prove that our watermarking scheme and one other scheme in the literature are, in fact, adaptively robust.”
Most existing technologies focus on making “zero-bit” watermarks, which can only embed a Yes/No signal into the output. In their paper, Cohen, Hoover, and Schoenbach use zero-bit watermarking schemes to construct a “message-embedding” watermark that embeds richer information, like a username or the timestamp of creation, into the outputs. For example, if a scammer were to use AI generated language to successfully scam someone out of their money, the message encoded in the watermark could help to identify the scammer.
Schoenbach noted that it was fairly simple for them to make the leap from a zero-bit watermarking to message-embedding scheme. However, the greater issue that the authors encountered was doing so in a general-purpose way, without relying on the specifics of any particular zero-bit scheme. Researchers prefer these “black-box” constructions, since future improvements made to the building blocks can be passed on to the larger construction. As Cohen noted, any improvements to the design of zero-bit schemes will automatically improve their black-box message-embedding scheme.
“The real challenge was figuring out how to come up with a unified language to describe what each of these watermarking schemes is doing,” Schoenbach chimed in. “Once we had that, it became much easier to write the paper.”
In order to build their message-embedding scheme, the authors needed to abstract away from existing zero-bit schemes. This ultimately took many iterations of drafting for them to fully understand, as they had to refine the concepts, their language, and also how they thought about the problem. Because their research is theoretical, they haven’t implemented their watermark, but hope to see their framework adopted in future watermarking schemes by other companies. Watermarking is becoming an increasingly urgent issue because of pressure from the federal government and the White House. Biden, specifically, has issued an executive order on ensuring “Safe, Secure, and Trustworthy Artificial Intelligence,” including through the use of watermarking and holding AI corporations accountable.
“AI companies don’t want to empower would-be abusers,” Cohen commented. “Whether they’re correctly balancing benefits and harms is something one could debate, but I think there is a genuine concern. At the very least, they want to mitigate harm to the extent that it doesn’t affect the quality of the outputs and doesn’t slow them down. It’s too soon to tell what role watermarking will have to play.? We’re in the very early days of this research, and the frontier of what is and isn’t possible we still don’t know, whether on the technical or policy side. ”
Currently, the authors are working on polishing and clarifying parts of their paper. To learn more about their research, please visit Cohen’s publication page here.