Yisong Yue (Caltech) - New Frontiers in Imitation Learning
New Frontiers in Imitation Learning
The ongoing explosion of spatiotemporal tracking & sensing data has now made it possible to analyze and model fine-grained behaviors in a wide range of domains. For instance, tracking data is now being collected for every NBA basketball game with players, referees, and the ball tracked at 25 Hz, along with annotated game events such as passes, shots, and fouls. Other settings include laboratory animals, people in public spaces, professionals in settings such as operating rooms, actors speaking and performing, digital avatars in virtual environments, phenomena in nature such as aerodynamics, and even the behavior of other computational systems.
In this talk, I will describe ongoing research in developing structured imitation learning approaches to develop predictive models of fine-grained behavior. Imitation learning is branch of machine learning that deals with learning to imitate dynamic demonstrated behavior. Structured imitation learning pertains imposing mathematically rigorous domain knowledge that can (sometimes provably) accelerate learning, and can also provide side benefits (such as Lyapunov stability or interpretability of policy behavior). I will provide a high level overview of the basic problem setting, as well as specific projects in modeling laboratory animals, professional sports, speech animation, and expensive computational oracles.
Host: Yuxin Chen
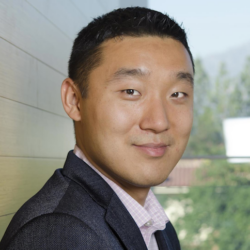
Yisong Yue
Yisong Yue is an assistant professor in the Computing and Mathematical Sciences Department at the California Institute of Technology. He was previously a research scientist at Disney Research. Before that, he was a postdoctoral researcher in the Machine Learning Department and the iLab at Carnegie Mellon University. He received a Ph.D. from Cornell University and a B.S. from the University of Illinois at Urbana-Champaign.
Yisong's research interests lie primarily in the theory and application of statistical machine learning. He is particularly interested in developing novel methods for interactive machine learning and structured machine learning. In the past, his research has been applied to information retrieval, recommender systems, text classification, learning from rich user interfaces, analyzing implicit human feedback, clinical therapy, tutoring systems, data-driven animation, behavior analysis, sports analytics, experiment design for science, learning to optimize, policy learning in robotics, and adaptive planning & allocation problems.